Research
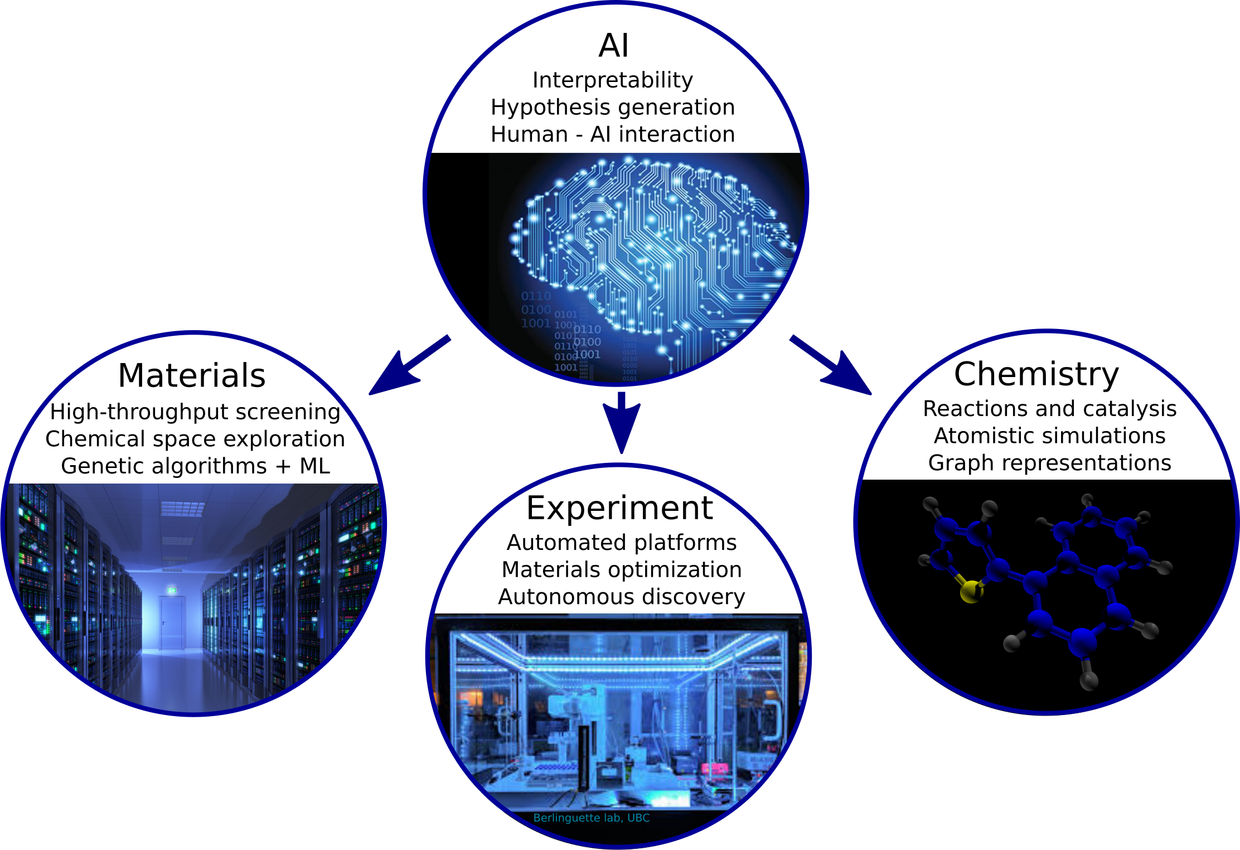
Research highlights
Machine-learned potentials for next generation matter simulations
The choice of simulation methods in computational materials science is driven by a fundamental trade-off: bridging large time- and length-scales with highly accurate simulations at an affordable computational cost. Venturing the investigation of complex phenomena on large scales requires fast yet accurate computational methods. We review the emerging field of machine-learned potentials, which promises to reach the accuracy of quantum mechanical computations at a substantially reduced computational cost. This Review will summarize the basic principles of the underlying machine learning methods, the data acquisition process and active learning procedures. We highlight multiple recent applications of machine-learned potentials in various fields, ranging from organic chemistry and biomolecules to inorganic crystal structure predictions and surface science. We furthermore discuss the developments required to promote a broader use of ML potentials, and the possibility of using them to help solve open questions in materials science and facilitate fully computational materials design.
Machine learning enables simplified measurement of material properties for future autonomous laboratories.
The automation of scientific experiments with artificial intelligence has the potential to significantly accelerate the development and optimization of novel materials with tailored properties [1].
In a recently published article [2] in ACS Nano, the authors show that they can use neural networks to draw conclusions about the conductivity of polymer mixtures from comparatively easy to measure optical properties. The conductivity of polymers is more interesting, but also much more difficult to measure than optical properties. This principle can also be transferred to other materials and properties. The use of artificial intelligence methods therefore has the potential to optimize the numerous parameters available for the development of materials in faster and more cost-effective way by replacing complex measurement methods for determining material properties with rapid, e.g. optical experiments, which then use machine learning to predict the actual target property.
[1] Mission Innovation: Materials Acceleration Platform, Report of the Clean Energy Materials Innovation Challenge Expert Workshop, January 2018
[2] Loïc M Roch, Semion K Saikin, Florian Häse, Pascal Friederich, Randall H Goldsmith, Salvador León, Alán Aspuru-Guzik, From Absorption Spectra to Charge Transfer in Nanoaggregates of Oligomers with Machine Learning, ACS Nano 2020
Design of new catalysts by artificial intelligence.
Catalysts are not only used to clean exhaust gases, but are also indispensable in the production of molecules and materials. The design of ever newer molecules and materials would be unthinkable without the simultaneous development of newer and better catalysts. In addition, novel catalysts also play a decisive role in recovering CO2 from the atmosphere and thus in combating climate change. A recent study [1] published in Chemical Science demonstrates how machine learning can be coupled with highly accurate but expensive simulation methods to predict the efficiency of new catalysts by computational methods and thus significantly accelerate their design. Pascal Friederich, newly appointed junior professor at KIT, and his co-authors show in the article that machine learning is not a black box but can be interpreted to derive design rules for new catalysts that are intelligible to scientists. The proposed method for the virtual design of new iridium catalysts can be transferred as desired to further classes of reactions of homogeneous (and in principle also heterogeneous) catalysis.